DeepMind’s AlphaGo defeats Go champion
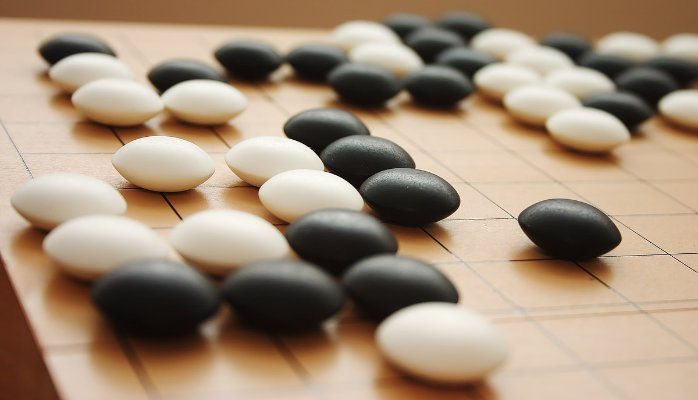
A few months ago, a computer program defeated a professional player of 2-dan rank, Fan Hui, known for having won the European Go championship three times in a row in 2013, 2014, and 2015. AlphaGo won 5-0 (normal games without a handicap), and 3-2 (time-controlled games without a handicap). This is obviously a significant milestone in the history of Go and in the field of machine learning, as Go is considered by many AI specialists as the most challenging of classic games, due to its enormous search space and the complexity of evaluating board positions and moves.During the match against Fan Hui, AlphaGo evaluated thousands of times fewer positions than Deep Blue did in its chess match against Kasparov in 1997, compensating by selecting those positions more intelligently, an approach that is probably closer to how humans play.
AlphaGo relies on deep neural networks and a particular 2-stage training pipeline combining supervised learning from human expert games and reinforcement learning from games of self-play. It introduces a new convolutional architecture supporting value networks to evaluate board positions and policy networks to select moves. To narrow the beam of the search tree to high-probability moves, a Monte-Carlo Tree Search algorithm is run asynchronously. Multiple simulations are executed in parallel on separate search threads. The current non-distributed version of AlphaGo uses 40 search threads, 48 CPUs, and 8 GPUs.
In March 2016, AlphaGo will face a legendary challenger, Lee Sedol, a top Korean player of 9-dan rank.
StartFragment
EndFragment