The ghost in the machine
- Eric Boillaud
- Mar 12, 2016
- 7 min read
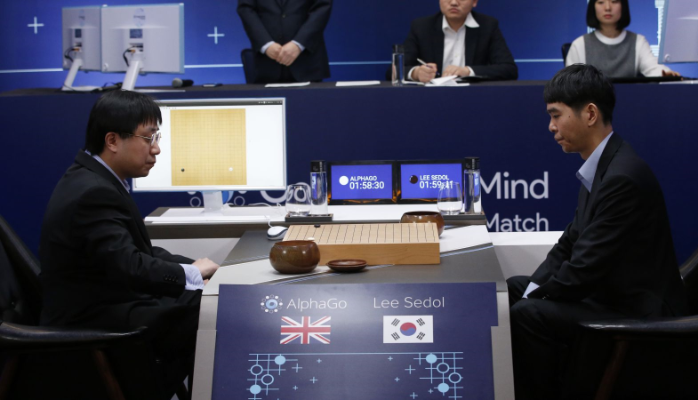
You’re probably going to read it in the newspaper or heard it on the TV or radio news today: a few hours ago, a machine defeated a world-class Korean Go champion, something that the majority of the world’s top players had thought to be practically impossible. Google’s artificial intelligence program AlphaGo just made AI history by scoring three victories in a row against Lee Sedol, a 9-dan professional Go player, undoubtedly one the world’s best players at the present time. I watched the latest of these three games live on Youtube tonight, and that was awesome. This is one more sign that we are entering the age of machine learning and of self-adaptive systems at an accelerated pace.Machine learning has already hit the market (search engines, online advertising, information retrieval, computer vision, speech recognition, natural language understanding, fraud detection, facial recognition, bioinformatics) and will continue doing so in the upcoming years (self-driving cars, service automation, health monitoring, medical diagnosis, robot locomotion). Our everyday life is going to change drastically, and personally I wouldn’t be surprised if machines were capable of writing poems or of composing music over a 10-year horizon. I would be amazed as I am now, but I would not be surprised. Yet, for many people, machine learning remains elusive. Let’s have a look at what AlphaGo’s victory really means.
Go, chess, and AI detractors
Go is a fascinating game, with an aesthetic that is minimalist to the extreme. This is also one of the most profound and engaging games that you could ever play. Go is played on a board marked with a 19x19 grid, the aim being to surround more territory than the opponent. This is a combinatorial game with perfect information, and the sheer amount of variations per move results in situations that are too vague to be calculable, even by a machine. When expressing their passion for Go, professional players sometimes explain that they feel like taking part in something much bigger and more important than a game. Subjectively speaking, I think it is an experience more akin to meditation, and at the same time closely related to high performance sport. In Korea, professional Go players are regarded with the same reverence as martial arts practitioners, and as such are deeply respected.
It’s been two decades since world chess champion Garry Kasparov was first defeated by a computer. And each time computers show their remarkable abilities, detractors claim that artificial intelligence remains inferior in essence to human reasoning, because it relies on brute-force algorithms that consist in methodically exploring every possible move instead of creatively converging toward a solution. I won’t waste time arguing that human chess and Go champions, as machines do, study and memorize thousands of sequences of moves encyclopedically. I’d rather argue, more interestingly, that self-adaptive systems do not rely on brute-force algorithms, and that instead they are capable of discovering by themselves patterns that make sense.
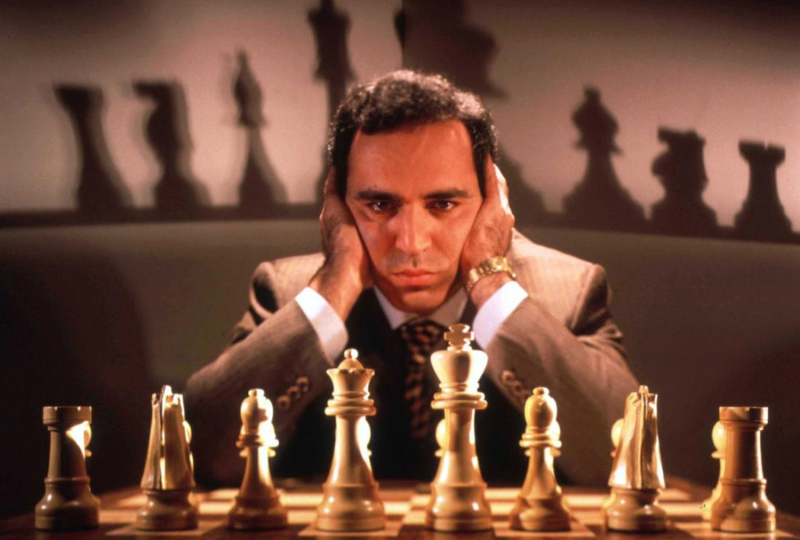
Machine learning: everything is a pattern
Machine learning is not something new. Actually it started five decades ago with the Perceptron and even earlier with first-generation statistical models. Machine learning is based on the simple idea that any type of intelligent system, artificial or natural, can be defined as a pattern recognizer, provided that such a system might be capable of learning how to probabilistically detect by itself the patterns that make sense in the context of the task it needs to accomplish. Animal intelligence has been evolving from this basic principle for millions of years. But it’s only in the past few years that AI practitioners made stunning achievements in mimicking natural intelligent systems, because they were only recently capable of leveraging huge storage capacities and computer processing power to experiment with tremendously sophisticated models.Whilst machine learning was at first limited to perceptual applications (such as recognizing letters, signatures, written words, or spoken words), it is now widely applied to semantic problems (such as mention detection and intent classification from naturally phrased expressions), or problem solving (such as question answering or in-game intelligence).
Machine learning is going to revolutionize the way we approach automation in multiple domains, because practically anything you can think of can be processed generically as a pattern. A concrete visual form is a pattern, which explains the success of the industrial neural networks in computer vision and automation in the 1990s. A concrete physical movement or control process is a pattern, which explains the imminent wave of self-driving cars and delivery drones. And more generally, any abstract form within a sequence of reasoning can also be regarded as a pattern. The consequences of the latter in the next two decades will be huge, as many researchers are already experimenting with new ways to interconnect hierarchically such abstract patterns for problem solving applications.
EndFragment

Can AI be creative?
Now let’s go back to Go. AlphaGo learns from experience game after game, and evaluates thousands of times fewer positions than Deep Blue did in its chess match against Kasparov in 1997, compensating by selecting those positions more intelligently. This approach is closer to how humans think, and this is exactly what machine learning is about. While reading this, you are probably wondering if machine learning would not finally relate to a particular form of artificial creativity. An interesting question is: did AlphaGo beat Lee Sedol because there was some creativity in its moves, or rather because Lee Sedol played a bad move at some point? Well, I would respond with another question: is it not the same thing after all? Or I could suggest, even more ambiguously, that AlphaGo forced Lee Sedol to play a bad move at some point. Or, more seriously, I could emphasize the concept of emergence, whereby creative patterns at higher scale arise through interactions among simpler patterns that themselves do not exhibit such properties. In any case, AlphaGo and Lee Sedol played three completely unique games that were never played before in the history of Go, and that tells something about creativity, or about adaptability at the very least.
Let's review these three games.
In the first game, Lee Sedol (playing black – i.e., going first) started with quite unorthodox moves. Perhaps he wanted to play an opening that AlphaGo was unlikely to have seen before. Lee Sedol played quite aggressively, and in response AlphaGo played thick and solid moves. AlphaGo managed to develop power in the center, while allowing Lee Sedol to gain territory on the right side. During the middlegame, AlphaGo demonstrated good skills at fighting. Not only did it maintain its position firmly, it also found opportunities for sharp counter-attacks on weak black stones. AlphaGo’s endgame proved excellent, and Lee Sedol eventually lost the advantage in the bottom right corner after AlphaGo managed to invade this side. Globally, AlphaGo showed very good positional judgement and timing.
The second game was totally different. Lee Sedol (playing white – i.e., going second) played more cautiously, but was forced to respond to an unusual opening from AlphaGo. Throughout the game, Lee Sedol consolidated his position at the top. AlphaGo played unexpected moves, some of them brilliant, others quite surprising, and managed to build up its framework while accommodating its opponent’s moves with much flexibility. Globally, AlphaGo proved capable of playing innovative moves and of taking control of the game. At the post-game press conference, Lee Sedol said: “Yesterday I was surprised, but today, more than that, I’m quite speechless.”
The third game, I already told you, was awesome. Lee sedol played black (which means he played the first move as in the first game). I could not tell who played offensively or defensively, as the two players seemed to do both things at the same time, especially on the left side where complicated battles were continuously fought. It was like watching a clash of titans, in slow motion, with no sound effect, but fueled with much caffeine (at least on my end, as Lee Sedol seemed more inclined to drinking tea and AlphaGo some kind of electric juice). Globally, AlphaGo’s victory was impressive. At the post-game press conference, Lee Sedol said: “I’ve never played a game where I felt this amount of pressure, and I wasn’t able to overcome this pressure.”
In conclusion, there is (obviously) no hardcoded creativity in AlphaGo. How could its designers have predetermined how and when to play innovative moves? But throughout these three games, some kind of creativity emerged spontaneously from AlphaGo’s play. If you doubt it, ask Lee Sedol.
Poker, and again, AI detractors
I admit being somewhat disappointed by the general tendency to ontologically disregard any progress made in AI. I think this is a form of denialism. As soon as machines are getting good at something, detractors dismiss the argument as a valid demonstration of real intelligence. Now I can almost imagine what they are going to say of AlphaGo's victory. If computers could beat us at poker, that would be a genuine example of artificial intelligence. But in order to beat us at poker, computers would need to interpret complex social behaviors such as risk-taking and bluffing, and they won’t be able to do that. At this point, however, that’s not a bet I would advise them to take. And in fact, I would tend to predict that the next big thing that is going to be successfully automated is precisely smarter pokerbots, and that they will soon beat the world’s best players. Machine learning is exceedingly good at discovering hidden correlations between variables that cannot be clearly defined as such, even when these variables are loosely merged in a multitude of events like in a poker game. The way that human players are likely to bet is a typical example of what machines are capable of learning. And because machines are also exceedingly good at computing the odds of any particular draw, they will be capable of adapting to human play. And as for reading the poker face of their opponent, why not? Facial interpretation is already in the pipe anyway.
You know what? After all, this is all a game, let’s bet!
EndFragment
EndFragment
StartFragment
EndFragment
Comments